Artificial Intelligence in medical practice: closing the gap for the present and creating opportunities for the future
DOI:
https://doi.org/10.60787/tnhj.v23i2.655Keywords:
the future, benefits, contemporary medical practice, Artificial intelligenceAbstract
Background: Artificial intelligence (AI) is being incorporated into every aspect of human endeavour with benefits in diverse ways. Its application has brought a revolutionary dimension to healthcare delivery services across the globe. The aim of this study is to appraise the concept of artificial intelligence as it is applicable to contemporary medical practice and also looking into opportunities to come in its future application of AI.
Method: This is a narrative review article on AI, in which literatures were searched on AI using PubMed, Google Scholar, and MEDLINE. The search keywords were artificial intelligence concerning the basic theory, clinical and non-clinical applications, and future medical and global economic benefits. A critical review of the article was then undertaken.
Results: Eight-seven articles were screened of which, 46 articles were found to be relevant for the review. Artificial Intelligence has been found useful across various specialties of medicine through its precision, error-reduction ability and prediction of clinical outcomes. Despite this, AI-driven practice is still at a rudimentary stage and likewise the knowledge about AI in sub-Saharan Africa with a paucity of researches and publications on AI.
Conclusion: The use of AI in medical practice is increasing rapidly and has a great propensity to revolutionize patient care through better precision and reduction of medical errors. Despite this, AI in medical practice is still in the infancy stage in sub-Saharan Africa, we thus suggest the need to move from a position of a spectator to that of an active participant by embracing this innovation
Downloads
References
Madhusudana GS, KolinP, Senthil K, Nirmal KG. Artificial Intelligence and Deep Learning: The Future of Medicine and Medical Practice. J Assoc Physicians India. 2019; 67(4):71-73.
Luxton DD. Ethical challenges of conversational agents in global public health. Bull World Health Organ. 2020; 98 (4): 285-287.
Esteva A, Robicquet A, Ramsundar B, Kuleshov V, DePristo M, Chou K, et al. A guide to deep learning in healthcare. Nat Med. 2019;25(1):24–9.
Topol EJ. High-performance medicine: the convergence of human and artificial intelligence. Nat Med. 2019;25(1):44-56. doi: 10.1038/s41591-018-0300-7.
Goodman K, Zandi D, Reis A, Vayena E. Balancing risks and benefits of artificial intelligence in the health sector. Bull World Health Organ. 2020; 98 (4): 230-230A. doi:10.2471/BLT.20.253823.
Eli-Chukwu NC. Applications of artificial intelligence in agriculture: A review. Eng. Technol. Appl. Sci. Res. 2019; 9(4): 4377-4383.
Hadlington L. Binder J, Gardner S, Karanika-Murray M, Knight S. The used of artificial intelligence in a military context: development of the attitudes towards AI in defense (AAID) scale. Front Psychol. 2023; 14: 1164810. doi:10.3389/fpsyg.2023.1164810.
Sahu A, Mishra J, Kushwaha N. Artificial intelligence (AI) in drugs and pharmaceuticals. Comb Chem High Throughput Screen. 2022; 25 (11): 1818-1837. doi: 10.2174/1386207325666211207153943
Davenport T, Kalakota R. The potential for artificial intelligence in healthcare. Future Healthc J. 2019; 6(2):94-98. doi: 10.7861/futurehosp.6-2-94. PMID: 31363513; PMCID: PMC6616181.
Padoy N, Blum T, Ahmadi SA, Feussner H, Berger MO, Navab N. Statistical modeling and recognition of surgical workflow. Med Image Anal. 2012;16(3):632–41.
LeCun Y, Bengio Y, Hinton G. Deep learning. Nature. 2015;521(7553):436–44.
Choy G, Khalilzadeh O, Michalski M, et al. Current Applications and Future Impact of Machine Learning in Radiology. 2018; Radiology 171820. doi: 10.1148/radiol.2018171820.
Urban G, Tripathi P, Alkayali T, Mittal M, Jalali F, Karnes W et al. Deep Learning Localizes and Identifies Polyps in Real Time with 96% Accuracy in Screening Colonoscopy. Gastroenterology. 2018; 155(4): 1069-1078. doi: 10.1053/j.gastro.2018.06.037.
Misawa M, Kudo S, Mori Y, Cho T, Kataoka S, Yamauchi A et al. Artificial Intelligence-Assisted Polyp Detection for Colonoscopy: Initial Experience. Gastroenterology. 2018; 154: 2027–2029.
Mori Y, Kudo S, Berzin TM, Misawa M, Takeda K. Computer-aided diagnosis for colonoscopy. Endoscopy.2017; 49: 813–9.
AtashzarSF, Patel RV. Teleoperation for minimally invasive robotics-assisted surgery. Biomed. Eng. 2018; 4: 341–372. doi:10.1142/9789813232266_0012.
Zemmar, A, Lozano AM, Nelson BJ. The rise of robots in surgical environments during COVID-19. Nat. Machine Intelligence. 2020; 2(10): 566–572. doi:10.1038/s42256-020-00238-2.
Gopichandran V, Ganeshkumar P, Dash S, Ramasamy A. Ethical challenges of digital health technologies. Bull World Health organ. 2020; 98(4): 277-281.
Halcox JPJ, Wareham K, Cardew A, Gilmore M, Barry JP, Phillips C, et al. Assessment of remote heart rhythm sampling using the AliveCor heart monitor to screen for atrial fibrillation: the REHEARSE-AF study. Circulation. 2017; 136:1784–94. doi: 10.1161/CIRCULATIONAHA.117.030583.
Turakhia MP, Desai M, Hedlin H, Rajmane A, Talati N,Ferris T, et al. Rationale and design of a large-scale, app-based study to identify cardiac arrhythmias using a smartwatch: the apple heart study. Ame Heart J. 2019; 207:66–75. doi: 10.1016/j.ahj.2018.09.002
Huang Z, Chan TM, Dong W. MACE prediction of acute coronary syndrome via boosted resampling classification using electronic medical records. J Biomed Inform. 2017; 66:161–70. doi: 10.1016/j.jbi.2017. 01.001.
Mortazavi BJ, Downing NS, Bucholz EM, Dharmarajan K, Manhapra A, Li SX, et al. Analysis of machine learning techniques for heart failure readmissions. Circ Cardiovasc Qual Outcomes. 2016; 9:629–40.doi: 10.1161/CIRCOUTCOMES.116.003039.
Regalia G, Onorati F, Lai M, Caborni C, Picard RW. Multimodal wrist-worn devices for seizure detection and advancingresearch: focus on the Empatica wristbands. Epilep Res. 2019; 153:79–82. doi: 10.1016/j.eplepsyres.2019.02.007.
Bruno E, Simblett S, Lang A, Biondi A, Odoi C, Schulze-Bonhage A, et al. Wearable technology in epilepsy: the views of patients, caregivers, and healthcare professionals. EpilepBehav. 2018; 85:141–9. doi: 10.1016/j.yebeh.2018.05.044.
Lawton J, Blackburn M, Allen J, Campbell F, Elleri D, Leelarathna L, et al. Patients’ and caregivers’ experiences of using continuous glucose monitoring to support diabetes self-management: qualitative study. BMC Endocrine Disord. 2018; 18:12. doi: 10.1186/s12902-018-0239-1.
Christiansen MP, Garg SK, Brazg R, Bode BW, Bailey TS, Slover RH, et al. Accuracy of a fourth-generation subcutaneous continuous glucose sensor. Diabet Technol Therapeut. 2017; 19:446–56. doi: 10.1089/dia.2017.0087.
da Silva DA, ten Caten CS, dos Santos RP, FogliattoFS, HsuanJ. Predicting the occurrence of surgical site infections using text mining and machine learning. PLOS ONE 14(12): e0226272. https://doi.org/10.1371/journal.pone.0226272
Poplin R, Varadarajan AV, Blumer K, Liu Y, McConnell MV, Corrado GS, et al. Prediction of cardiovascular risk factors from retinal fundus photographs via deep learning. Nat Biomed Eng. 2018;2(3):158–64.
Esteva A, Kuprel B, Novoa RA, Ko J, Swetter SM, Blau HM, et al. Dermatologist-level classification of skin cancer with deep neural networks. Nature. 2017;542(7639):115–8
McKinney SM, Sieniek M, Godbole V, Godwin J, Antropova N, Ashrafian H, et al. International evaluation of an AI system for breast cancer screening. Nature. 2020; 577(7788):89–94.
Maier-Hein L, Vedula SS, Speidel S, Navab N, Kikinis R, Park A, et al. Surgical data science for next-generation interventions. Nat Biomed Eng. 2017;1(9):691–6.
van Roessel S, Strijker M, Steyerberg EW, Groen JV, Mieog JS, Groot VP, et al. International validation and update of the Amsterdam model for prediction of survival after pancreatoduodenectomy for pancreatic cancer. Eur J Surg Oncol. 2019:105455.
Basta MN, Kozak GM, Broach RB, Messa CA, 4th, Rhemtulla I, DeMatteo RP, et al. Can We Predict Incisional Hernia? Development of a Surgery-specific Decision-Support Interface. Ann Surg. 2019; 270(3):544–53.
Hechi ME, Gebran A, Bouardi HT, Maurer LR, Moheb ME, Zhuo D et al. Validation of the artificial intelligence–based trauma outcomes predictor (TOP) in patients 65 years and older. SURGERY.2022;171(6): 1687–1694. doi: 10.1016/j.surg.2021.11.016
Lalys F, Jannin P. Surgical process modelling: a review. Int J CARS. 2014; 9(3):495–511.
Tokuyasu T, Iwashita Y, Matsunobu Y, Kamiyama T, Ishikake M, Sakaguchi S, et al. Development of an artificial intelligence system using deep learning to indicate anatomical landmarks during laparoscopic cholecystectomy. Surg Endosc. 2020 :1–8.
Twinanda AP, Shehata S, Mutter D, Marescaux J, de Mathelin M, Padoy N. Endonet: A deep architecture for recognition tasks on laparoscopic videos. IEEE Trans Med Imaging. 2017; 36(1):86–97.
Smith MJ, Axler R, Bean S, Rudzicz F, Shaw J. Four equity considerations for AI in public health. BullWorld Health organ. 2020; 98(4): 290-292.
Paul AK, Schaefar M. The real safeguard for artificial intelligence and machine learning in global health systems. Bull World Health organ. 2020; 98(4): 282-284.
Bogue, R. Robots in a contagious world. Industrial Robot. 2020; 47 (5): 673–642. doi:10.1108/IR-05-2020-0101.
Panesar S, Cagle Y, Chander D, Jose Fernandez-Miranda, J, Kliot M. Artificial Intelligence and the Future of Surgical Robotics. Annals of Surgery.2019; 270 (2): 223-226. doi: 10.1097/SLA.0000000000003262.
Hademan A, Decker RS, Opfermann JD, Leonard S, Krieger A, Kim PC. Supervised autonomous robotic soft tissue surgery. Sci Transl Med. 2016;8(337):337ra64.
Yao Y, Zhou Y, Liu L, Xu Y, Chen Q, Wang Y et al. Nanoparticle-based drug delivery in cancer therapy and its role in overcoming drug resistance (Review article). Front. Mol. Biosci. 7:193. doi: 10.3389/fmob.2020.00193.
Acharya S, Sahoo SK. PLGA nanoparticles containing various anti-cancer agents and tumour delivery by EPR effect. Adv Drug Deliv. Rev.63:170-183. doi: 10.1016/j.addr.2010.10.008.
Yu Z, Gao L, Chen K, Zhang W, Zang Q, Li Q et al. Nanoparticles: a new approach to upgrade cancer diagnosis and treatment. Nanoscale Res Lett. 2021; 16:88.
Acampora G, Cook DJ, Rashidi P, Vasilakos AV. A survey on ambient intelligence in healthcare.Proc IEEE Inst Elect Electron Eng. 2013; 101: 2470-94. doi:10.1109/JPROC.2013.2262913.
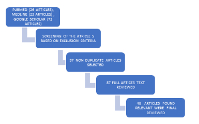
Downloads
Published
How to Cite
Issue
Section
License
Copyright (c) 2023 Journal and Publisher

This work is licensed under a Creative Commons Attribution-NonCommercial-NoDerivatives 4.0 International License.
The Journal is owned, published and copyrighted by the Nigerian Medical Association, River state Branch. The copyright of papers published are vested in the journal and the publisher. In line with our open access policy and the Creative Commons Attribution License policy authors are allowed to share their work with an acknowledgement of the work's authorship and initial publication in this journal.
This is an open access journal which means that all content is freely available without charge to the user or his/her institution. Users are allowed to read, download, copy, distribute, print, search, or link to the full texts of the articles in this journal without asking prior permission from the publisher or the author.
The use of general descriptive names, trade names, trademarks, and so forth in this publication, even if not specifically identified, does not imply that these names are not protected by the relevant laws and regulations. While the advice and information in this journal are believed to be true and accurate on the date of its going to press, neither the authors, the editors, nor the publisher can accept any legal responsibility for any errors or omissions that may be made. The publisher makes no warranty, express or implied, with respect to the material contained herein.
TNHJ also supports open access archiving of articles published in the journal after three months of publication. Authors are permitted and encouraged to post their work online (e.g, in institutional repositories or on their website) within the stated period, as it can lead to productive exchanges, as well as earlier and greater citation of published work (See The Effect of Open Access). All requests for permission for open access archiving outside this period should be sent to the editor via email to editor@tnhjph.com.